Fuel energy resilience and reinvention
Demand is up. Competition is fierce. People expect sustainability. With inflationary pressures, supply chain network disruptions, and an increased focus on security, energy companies need to change today to compete tomorrow.
Energy now
50%
the estimated growth in demand for secure and sustainable energy over the next three decades as global GDP and populations grow
48%
of executives expect their organization to primarily benefit from investments in AI in terms of improved effectiveness
99%
of energy executives agree investments in emerging technologies will help their organizations remain resilient on the global stage
97%
of energy executives agree the convergence of digital and physical worlds over the next decade will transform their industry
How to reinvent energy
Accelerate towards a net-zero emissions future
Make smarter, faster decisions
Protect your digital assets and ecosystem
Data and digital technologies to increase competitiveness and resiliency
A holistic approach to compete in a volatile world
Empower your workforce
Enable optimization of commercial and supply chain decisions
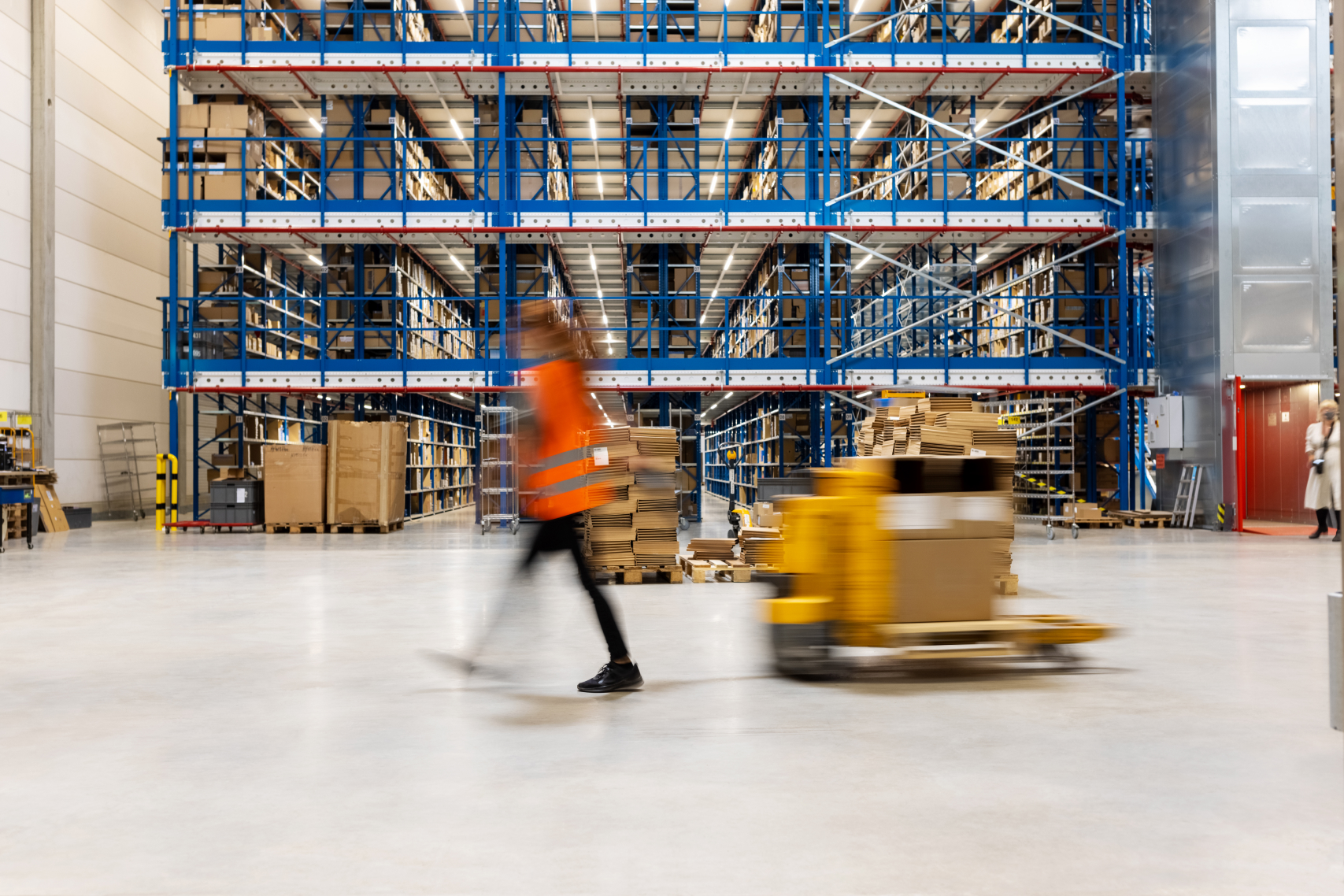
Keep up with customer expectations and a competitive landscape
Integrated, intelligent solutions toward total enterprise reinvention
Create a robust portfolio for growth and energy transition to net-zero
What’s trending in energy
Awards and recognition
Our leaders
John Downie
Senior Managing Director – Strategy & Consulting, Global Energy Lead
Herve Wilczynski
Managing Director – Strategy & Consulting, Energy Upstream Lead
Andrew Smart
Senior Managing Director – Strategy & Consulting, Energy Lead, EMEA
Paul Carthy
Managing Director – Strategy & Consulting, Energy Lead, Growth Markets